In an era where cyber threats are becoming increasingly sophisticated, leveraging AI for endpoint security is essential—but not without challenges. Discover how to enhance your security posture while safeguarding sensitive data and maintaining compliance.
Data privacy in AI-driven endpoint security is crucial for protecting sensitive information. AI enhances security through continuous monitoring, behavioral analysis, and advanced threat detection, but it also brings risks.
Data poisoning and model inversion attacks can compromise your data privacy, making robust security measures essential. To safeguard your sensitive information, implement strict access controls, utilize multi-factor authentication, and foster a culture of security awareness among employees.
Regular assessments help identify vulnerabilities and counter evolving threats. By understanding these dynamics, you can better secure your systems against breaches and privacy violations while leveraging AI’s advantages. Discover more effective strategies for protection.
Key Takeaways
- AI enhances endpoint security but introduces data privacy risks like data poisoning and model inversion attacks.
- Implement strong encryption, multi-factor authentication, and employee training to mitigate these risks effectively.
- Compliance with data regulations such as GDPR and HIPAA is crucial when integrating AI into security systems.
- Balancing innovation with vigilance ensures you leverage AI benefits while protecting sensitive information.
Overview of AI and Data Security
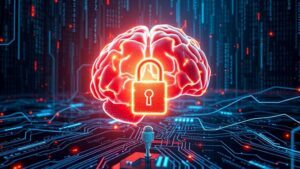
AI greatly enhances security by analyzing vast amounts of data to identify and respond to threats in real time.
However, this reliance on data also raises critical vulnerability concerns, as sensitive information could be exposed to cyber threats.
You’ll need to navigate the complexities of privacy compliance to guarantee that the benefits of AI don’t compromise data protection standards.
AI Enhancements in Security
In today’s digital landscape, integrating advanced technologies into endpoint security is becoming increasingly crucial for organizations. AI technology plays a fundamental role in enhancing your security posture by improving threat detection and response capabilities. By analyzing vast data sets in real-time, AI can identify patterns and anomalies that traditional methods often miss.
Consider these key benefits of AI enhancements in security:
- Behavioral Analysis: AI solutions continuously monitor user and device activities, flagging deviations that may indicate a real-time threat.
- Advanced Threat Detection: Techniques like deep learning help identify complex malware variants, including polymorphic malware and fileless attacks.
- Continuous Improvement: Reinforcement learning allows AI algorithms to adapt to evolving threats, enhancing detection efficacy and minimizing false positives over time.
With these capabilities, organizations can considerably reduce the risk of data breaches while maintaining data privacy.
Leveraging AI-driven endpoint security solutions not only strengthens defenses but also fosters a proactive approach to cybersecurity. As cyber threats grow more sophisticated, integrating AI becomes crucial for maintaining robust security measures and ensuring compliance with data protection regulations.
Data Vulnerability Concerns
Data vulnerability concerns in the domain of endpoint security have become increasingly prominent as organizations integrate AI technologies. While AI systems enhance security measures, they also introduce significant risks, particularly regarding data privacy. As these systems rely heavily on data for training and operation, they become prime targets for malicious actors seeking to exploit vulnerabilities.
One major threat is data poisoning, where attackers manipulate training data to alter the AI’s behavior. This can lead to privacy breaches and the dissemination of incorrect outputs. Additionally, model inversion attacks can reverse-engineer sensitive training data, exposing personal information and violating compliance with regulations such as GDPR and HIPAA.
To mitigate these risks, organizations must implement robust endpoint security measures, including encryption and multi-factor authentication, while fostering a culture of security awareness among employees.
The integration of AI in endpoint security necessitates ongoing efforts to protect sensitive information from data breaches and unauthorized access. Balancing the benefits of AI with stringent data protection protocols is essential to guarantee compliance and safeguard data privacy in an increasingly digital landscape.
Privacy Compliance Challenges
Maneuvering the complexities of privacy compliance presents significant challenges for organizations leveraging AI in endpoint security. As you integrate AI systems, you need to guarantee that your data collection and processing practices comply with regulations like GDPR and HIPAA.
The increase in sensitive data processed heightens the risk of data breaches, making it essential to implement robust protective measures.
To effectively address privacy challenges, consider the following strategies:
- Anonymization and Pseudonymization: Protect personally identifiable information in your AI training datasets.
- Continuous Monitoring: Regularly audit AI systems to identify and mitigate biases that could compromise data integrity.
- Adapting to Evolving Threats: Update your data privacy strategies to safeguard against adversarial attacks exploiting vulnerabilities in AI-driven systems.
Threats to AI Data Security
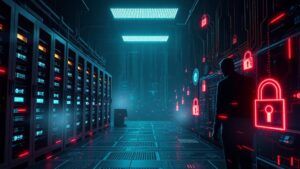
As you explore the threats to AI data security, it’s essential to take into account machine learning vulnerabilities that can be exploited by attackers.
Data breach risks also escalate as sensitive information may be exposed through weaknesses in AI models and systems.
Additionally, insider threats pose significant challenges, as individuals with access can manipulate data or systems for malicious purposes, undermining overall security.
Machine Learning Vulnerabilities
In the domain of endpoint security, machine learning vulnerabilities present substantial threats to AI data security. Understanding these vulnerabilities is essential for maintaining data privacy in an increasingly automated environment.
- Data poisoning can skew AI outcomes by manipulating training data, leading to ineffective security measures.
- Model inversion attacks allow attackers to reverse-engineer models and extract sensitive information, creating significant privacy risks.
- Adversarial attacks exploit minor perturbations in input data, deceiving models and potentially causing incorrect predictions.
The reliance on vast datasets for training machine learning systems heightens the risk of data breaches, as sensitive information can be exposed or misused.
Continuous model updates are imperative to combat these evolving threats, ensuring algorithms remain effective against new attack vectors.
Organizations should prioritize robust endpoint security measures, including encryption and multi-factor authentication, to safeguard against these machine learning vulnerabilities.
By fostering a culture of security awareness and implementing stringent privacy protocols, you can better protect sensitive data and maintain compliance with regulations.
In this landscape, recognizing and addressing these vulnerabilities is essential for sustaining the integrity of AI-driven security systems.
Data Breach Risks
The risks of data breaches in AI systems pose significant threats to data security, particularly as organizations increasingly rely on machine learning for endpoint protection. Data breaches can occur through various attack vectors, with malicious actors employing tactics like data poisoning to manipulate training data. This compromises the AI’s behavior and effectiveness, leading to severe consequences.
Model inversion attacks further exacerbate privacy risks, allowing unauthorized retrieval of sensitive training data, which can endanger individuals’ information. Additionally, adversarial attacks manipulate inputs to AI models, resulting in incorrect outputs that may expose sensitive data or compromise system integrity.
While insider threats can exploit access to AI systems, increasing the risk of unauthorized data exposure, it’s vital to understand that robust security frameworks are essential. Cyber threats targeting AI data can lead to significant financial and reputational damage for organizations.
To mitigate these risks, you need to prioritize thorough security measures, such as encryption and multi-factor authentication. By fostering a culture of security awareness and balancing the benefits of AI with stringent privacy protocols, you can better protect your organization against the ever-evolving landscape of data breaches.
Insider Threats
Insider threats represent a considerable challenge to AI data security, arising from both intentional and unintentional actions of employees who handle sensitive information. These threats can lead to data breaches and the manipulation of AI models, making it essential for organizations to implement robust security measures.
To effectively mitigate insider threats, consider the following strategies:
- Implement strict access controls to limit employee access to sensitive data and AI systems.
- Establish continuous monitoring to detect any suspicious activities or deviations from normal behavior.
- Provide regular employee training on data protection practices to raise awareness about the risks associated with mishandling sensitive information.
Data poisoning is a particularly concerning insider threat, where malicious insiders tamper with training datasets, altering AI behavior and potentially causing considerable harm.
Adopting a Zero Trust security model can further enhance your defense, as it guarantees that user identities are continuously verified and access is limited to only what’s necessary.
Securing AI Models
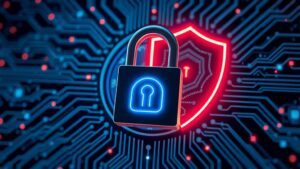
To effectively secure AI models, you need to focus on protecting the training data, implementing strict access control mechanisms, and conducting regular vulnerability assessments.
By isolating training environments, you can minimize the risk of unauthorized access and data leaks.
Additionally, establishing robust authentication protocols guarantees that only authorized personnel can interact with sensitive AI functionalities, safeguarding your organization’s data integrity.
Model Training Data Protection
In securing AI models during the training phase, organizations must prioritize creating isolated and controlled environments to prevent unauthorized access and data leaks.
This approach is essential for effective model training data protection, ensuring sensitive information remains secure throughout the process.
To enhance the security of AI models, consider implementing the following strategies:
- Conduct thorough data validation checks to identify irregularities that could lead to compromised model integrity.
- Utilize encryption for data, both in transit and at rest, to safeguard sensitive information against potential data breaches.
- Regularly assess AI systems for vulnerabilities and threats, enabling timely identification and mitigation of risks.
Access Control Mechanisms
Securing AI models requires robust access control mechanisms to guarantee that only authorized personnel can interact with sensitive training and deployment environments. These mechanisms are essential for preventing unauthorized access and potential data breaches that could compromise sensitive information.
Access Control Method | Description | Benefits |
---|---|---|
Multi-Factor Authentication | Requires users to provide multiple verification factors | Enhances security beyond just passwords |
Role-Based Access Control (RBAC) | Limits access based on user roles | Minimizes insider threats |
Continuous Monitoring | Tracks access logs and user activities | Detects unusual patterns for quick response |
Encryption | Protects data in transit and at rest | Safeguards against unauthorized access |
Implementing multi-factor authentication greatly boosts security by adding layers of verification. Role-based access control (RBAC) guarantees that only necessary personnel can access AI models, reducing risks associated with insider threats. Continuous monitoring of user activities allows you to quickly identify suspicious behavior, while encryption keeps sensitive information safe from prying eyes during interactions with AI models. By integrating these access control mechanisms, you can effectively fortify your AI infrastructure against potential vulnerabilities.
Vulnerability Assessment Strategies
Vulnerability assessments play an essential role in maintaining the integrity and security of AI models. By regularly evaluating your AI systems for weaknesses, you can proactively address potential threats before they become significant issues. This process is critical for guaranteeing data integrity and protecting sensitive information.
Consider these strategies for effective vulnerability assessments:
- Data Validation: Conduct thorough checks for irregularities in training data to guarantee its quality and reliability.
- Access Restrictions: Implement strict access controls in training environments to minimize unauthorized manipulation of AI models.
- Anomaly Detection: Use real-time monitoring systems to flag unexpected outputs, allowing for quick identification and mitigation of vulnerabilities.
Integrating cloud-based security measures further enhances your protection against external threats during the training and deployment phases.
By maintaining a vigilant approach to vulnerability assessments, you create a robust framework that safeguards your AI models. This not only secures sensitive data but also fosters trust in your systems, guaranteeing compliance with data privacy regulations.
Engage in these practices to fortify your endpoint security and protect against malicious exploitation effectively.
Best Practices for Data Privacy
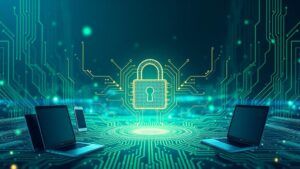
To guarantee data privacy in endpoint security, you need to implement strong encryption protocols and utilize multi-factor authentication effectively.
Regularly updating your security policies will keep your defenses robust against evolving threats.
Additionally, conducting employee training sessions fosters a culture of awareness that’s essential for safeguarding sensitive information.
Implement Strong Encryption Protocols
Implementing strong encryption protocols is essential for protecting sensitive data in today’s digital landscape. By utilizing robust encryption methods, you can greatly minimize the risk of unauthorized access to your information. Strong encryption protocols, such as AES-256, provide a solid foundation for maintaining data privacy, whether your data is at rest or in transit.
Consider the following best practices:
- End-to-End Encryption: This guarantees that only the communicating users can read the messages, safeguarding data from breaches.
- Regularly Update Encryption Algorithms: Staying current with the latest encryption algorithms is important, as outdated protocols may be susceptible to new attack vectors exploited by cybercriminals.
- Utilize Transport Layer Security (TLS): Implementing TLS for data transmission protects sensitive information from interception, securing communications across your endpoints.
Moreover, encrypting data backups is essential for maintaining data integrity and confidentiality, preventing cybercriminals from exploiting unsecured backups.
Utilize Multi-Factor Authentication
In today’s digital landscape, utilizing Multi-Factor Authentication (MFA) is essential for safeguarding sensitive data against unauthorized access. By requiring users to verify their identity through multiple methods, MFA can reduce the risk of breaches by 99.9% according to Microsoft.
To effectively implement MFA, use at least two of the three authentication factors: something the user knows (like a password), something the user has (such as a mobile device), and something the user is (biometric verification).
Ensure that all sensitive applications and data storage are protected by MFA, particularly in environments handling personal identifiable information (PII) to meet compliance with regulations like GDPR and HIPAA.
Regularly updating authentication methods and educating users on recognizing phishing attempts strengthens your security posture and enhances data privacy.
Consider adopting adaptive MFA, which tailors the authentication process based on user behavior and risk levels. This approach maintains robust security without compromising user experience.
Regularly Update Security Policies
While the digital landscape evolves at a rapid pace, staying ahead of emerging threats requires organizations to regularly update their security policies. This practice is essential not just for compliance with regulations like GDPR and HIPAA but also for enhancing data protection in AI systems.
By incorporating best practices into your security policies, you can greatly reduce the risk of data breaches.
Consider these key actions:
- Implement data anonymization and encryption to safeguard sensitive information.
- Establish a formal review schedule for policies to adapt to new technological advancements.
- Continuously monitor security measures to identify potential vulnerabilities, especially those affecting AI-driven systems.
Regular updates to your security policies foster a culture of data privacy and guarantee that your team remains informed about the latest threats.
Employee training plays a critical role in this process, equipping staff with the knowledge needed to recognize and respond to security challenges.
Conduct Employee Training Sessions
Regular updates to security policies lay the groundwork for a proactive approach to data privacy, but without thorough employee training, these policies may fall short. Conducting effective employee training sessions is essential in considerably reducing the risk of data breaches, as studies indicate that up to 90% of cybersecurity incidents stem from human error.
Your training should emphasize best practices, such as recognizing phishing attempts, managing passwords securely, and understanding the importance of data encryption. These elements are fundamental for protecting sensitive information.
Schedule regular training sessions—at least twice a year—to reinforce data privacy concepts and keep employees informed about the latest threats and compliance requirements, like GDPR and HIPAA.
Incorporating real-world scenarios and hands-on exercises can greatly enhance engagement and retention, making employees more adept at identifying and responding to potential security threats.
Additionally, providing resources such as quick-reference guides and ongoing support empowers employees to adhere to data privacy protocols. By fostering a culture of security awareness, you create an environment where everyone plays a role in safeguarding your organization’s data against breaches and cyber threats.
Regulatory and Ethical Considerations
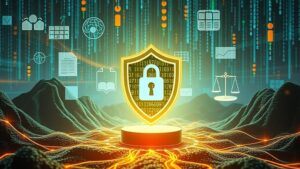
As you navigate the integration of AI in endpoint security, compliance with data regulations like GDPR and HIPAA becomes critical to avoid severe penalties.
You also need to adopt ethical AI usage standards, ensuring your algorithms don’t perpetuate bias.
Striking a balance between privacy and security is essential for maintaining consumer trust while effectively protecting sensitive information.
Compliance With Data Regulations
Maneuvering through the complexities of compliance with data regulations is essential for organizations leveraging AI in endpoint security. You must guarantee that your AI systems align with standards like GDPR and HIPAA to protect personal data and maintain user trust. This involves implementing transparent data processing practices and obtaining explicit consent from users, as mandated by regulations such as CCPA.
To effectively navigate compliance, consider the following:
- Data Protection by Design: Integrate privacy considerations into AI development from the start, guaranteeing that data protection is a fundamental aspect of your systems.
- Regular Audits: Conduct audits to identify and mitigate biases in AI systems, fostering fairness and accountability in alignment with ethical AI practices.
- Understand Consequences: Be aware that failure to comply with data protection regulations can lead to significant fines, with GDPR violations costing up to 4% of your annual global turnover.
Ethical AI Usage Standards
Steering compliance with data regulations sets the stage for establishing ethical AI usage standards, which are crucial in guaranteeing responsible data handling and decision-making. You must recognize that adhering to regulations like GDPR and HIPAA is critical for AI systems. This guarantees that personal data is collected, processed, and stored with transparency and accountability.
To promote ethical standards, organizations should implement clear data protection policies that prioritize user privacy and enhance consumer trust. Regular audits are necessary to identify and mitigate biases in AI algorithms, preventing discrimination and fostering fairness in decision-making.
The concept of explainable AI is crucial here, as it enables you and other stakeholders to understand how AI systems make decisions, reinforcing transparency.
Additionally, adopting a Zero Trust security framework guarantees continuous verification of access, minimizing risks associated with insider threats. This approach aligns with ethical considerations in data handling, reinforcing your organization’s commitment to compliance and data protection.
Balancing Privacy and Security
How can organizations effectively balance privacy and security in the age of AI?
It’s essential to navigate the complex landscape of data privacy while harnessing the power of AI and machine learning. By adhering to compliance regulations such as GDPR and HIPAA, you can guarantee responsible data handling that protects user privacy.
Regular audits can identify biases in AI systems, promoting ethical AI practices that foster fair decision-making.
Consider implementing the following strategies:
- Data Protection Techniques: Use anonymization and pseudonymization to safeguard personally identifiable information while allowing AI systems to operate effectively.
- Transparency and Control: Adhere to regulations like the California Consumer Privacy Act (CCPA), granting individuals greater control over their personal information.
- Security Awareness Culture: Foster a culture of security awareness within your organization, emphasizing the importance of safeguarding against security threats.
Balancing AI Innovation with Data Privacy—Securing Your Endpoint Protection
Incorporating AI into endpoint security doesn’t mean sacrificing data privacy. While some may worry about the risks, adopting robust protection measures like encryption and multi-factor authentication can effectively mitigate these concerns. By fostering a security-aware culture, your team can harness AI’s benefits while maintaining compliance with regulations. Remember, the goal isn’t just to protect data but to cultivate trust in your organization’s security practices. Balancing innovation with vigilance is key to safeguarding sensitive information.
Ready to enhance your organization’s security while maintaining strict data privacy? Don’t let data privacy concerns hold you back from leveraging the power of AI in your endpoint security.
Questions? We Have Answers.
Get answers to a list of the most Frequently Asked Questions.
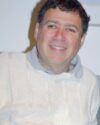
Experienced cybersecurity analyst, software engineer, patent attorney, worked with Linux, Windows, AWS, lots of security tools. Hope to help people do the right things and do the things right!